This content is for informational and entertainment purposes only, not financial advice. The authors are not professional traders. Trading involves risk and is not suitable for all investors. Decisions should be made with consideration from a financial advisor. This article may contain affiliate links. For full details, see our Affiliate Disclosure and Full Disclaimer.
Table of Contents
I. Introduction to Algorithmic Trading for Day Traders
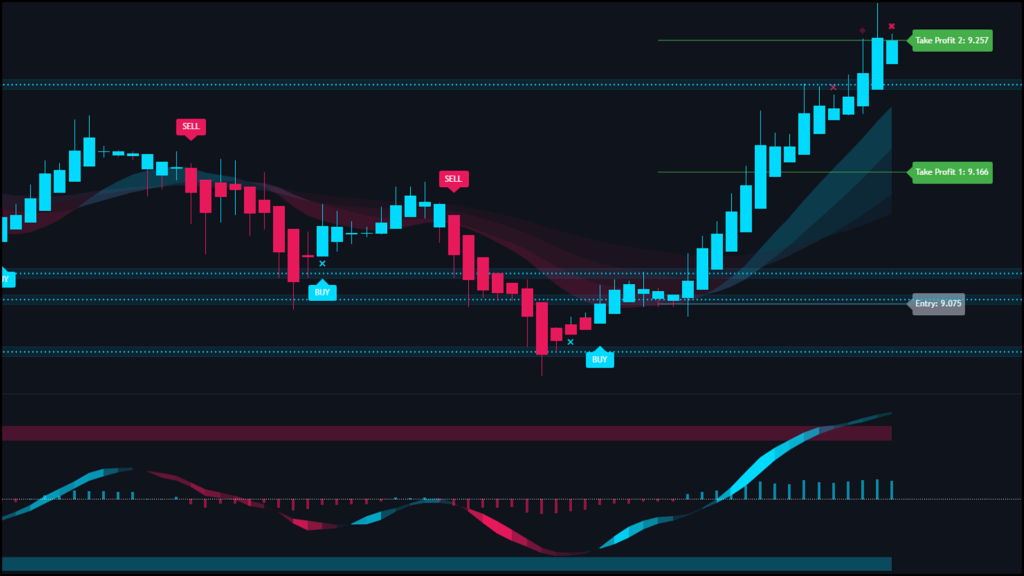
A. Market Evolution and Opportunities
In the dynamic realm of financial markets, algorithmic trading has emerged as a revolutionary force, reshaping the landscape of day trading. With its roots in sophisticated computer programs, algo trading enables traders to execute orders at unprecedented speeds and accuracy. This technological leap has particularly enhanced opportunities in day trading options for high-volume stocks like SPY and QQQ. As the market continues to evolve, it opens new avenues for traders to capitalize on minute price movements, making day trading more efficient and potentially more profitable.
B. Importance for Day Traders
For day traders, the importance of algorithmic trading cannot be overstated. In the fast-paced world of stock trading, where decisions must be made in fractions of a second, algo trading stands out as a crucial tool. It’s particularly relevant for popular stocks such as TSLA, AAPL, and META, where market movements can be rapid and unpredictable. By leveraging algorithms, day traders can execute complex trading strategies that respond quickly to market changes, ensuring they stay one step ahead in the competitive arena of stocks like AAPL, TSLA, and META. This approach not only enhances the potential for profit but also helps in effectively managing risk, making algorithmic trading an indispensable part of modern day trading strategies.
II. Understanding Algorithmic Trading: Basics for Beginners
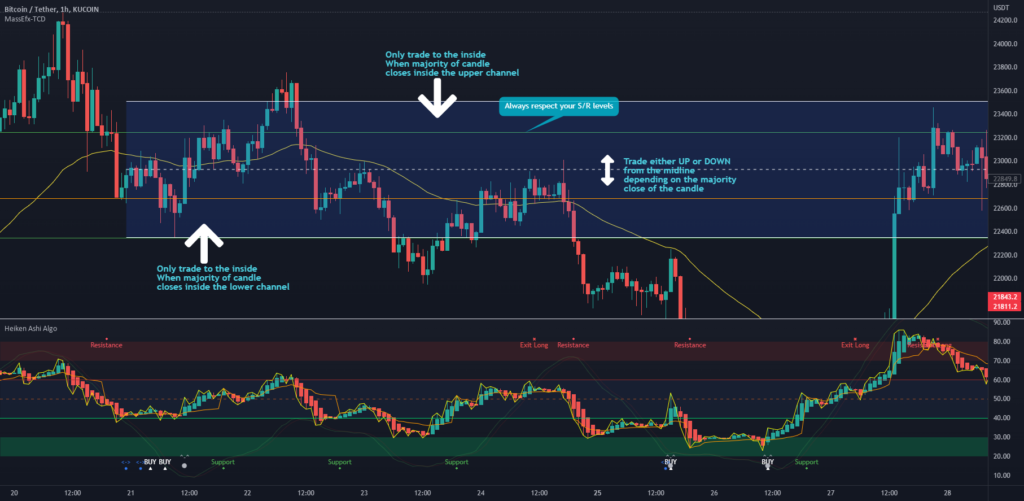
A. Algorithmic Trading Explained
Algorithmic trading, often referred to as algo trading, is the automated execution of trades using computer programs. These programs follow a defined set of instructions, or algorithms, to place trades at high speeds and volumes. This method is particularly advantageous in day trading, where it helps in capitalizing on small price fluctuations quickly. Algo trading removes emotional decision-making and ensures precision, making it a game-changer for beginners looking to step into the world of day trading.
B. Key Components of Algo Trading Systems
Key components of algo trading systems include advanced software algorithms, real-time market data, and a robust computational infrastructure. A prime example is EZAlgo, a trading algorithm that simplifies decision-making for day traders. It analyzes market data, identifies potential trading opportunities, and executes trades based on predefined criteria. For day traders, especially those trading in fast-moving markets like options, such tools are invaluable. They enable traders to efficiently manage multiple positions, react to market movements swiftly, and optimize their trading strategies, all while minimizing the risk of human error.
III. Navigating Key Trends in Algorithmic Trading for 2024

The landscape of algorithmic trading is being reshaped by several key technological trends as we move into 2024. These trends are not only transforming the sector but also offering new opportunities and challenges.
A. Technological Advancements in Algorithmic Trading
Intelligent Automation: A significant trend is the rise of intelligent automation, which is revolutionizing financial services by offering faster, more efficient, and accurate services. This technology is transforming the core processes in finance, setting new standards for operational efficiency.
AI and Machine Learning: AI and ML are significantly influencing personal finance management, with AI-driven financial advisors becoming increasingly common. These tools, employing advanced analytics and predictive modeling, are providing customized financial recommendations, enhancing decision-making in algorithmic trading.
Generative AI in Fintech: Generative AI models are being used in various fintech solutions, including algorithmic trading. They play a role in fraud prevention, asset management, and credit scoring, offering more nuanced and sophisticated analysis and decision-making capabilities.
Big Data and Analytics: The role of big data in the financial services industry is growing, with companies leveraging extensive datasets to gain insights into market trends and customer behavior. This allows for more informed trading strategies and improved operational efficiency.
Embedded Financial Solutions: The integration of financial services into various digital platforms, like mobile apps and websites, is making financial tools more accessible. This trend also includes the incorporation of trading tools and financial analysis into everyday digital solutions, enhancing accessibility for a broader range of users.
Each of these trends contributes to a more sophisticated and efficient environment for algorithmic trading, providing traders with enhanced tools and data to make informed decisions. As we progress into 2024, these technologies will continue to evolve, further shaping the landscape of algorithmic trading.
B. Impact on Day Trading Strategies
The aforementioned technological advancements are having a profound impact on day trading strategies in several ways:
Enhanced Decision-Making: With AI and ML, traders can process and analyze vast amounts of market data more quickly and accurately than ever before. This leads to more informed and strategic decision-making in real-time trading scenarios.
Automated and Customized Trading: Intelligent automation and generative AI enable the creation of personalized trading strategies. These technologies can automate routine trading tasks, adapt to new information, and execute trades based on predefined criteria, thus increasing efficiency.
Data-Driven Insights: Big data analytics provides traders with deeper insights into market trends and consumer behavior, enabling them to anticipate market movements more accurately and adjust their trading strategies accordingly.
Increased Accessibility: Embedded financial solutions are making advanced trading tools more accessible to a wider audience, including those new to day trading. This democratization of trading tools allows novice traders to engage in algorithmic trading with greater ease.
Overall, these technological trends are not only refining existing trading strategies but are also introducing new methodologies in day trading, leading to more dynamic and flexible approaches to navigating the financial markets.
IV. Essential KPIs for Evaluating Day Trading Performance

In the world of day trading, especially when using algorithmic strategies, tracking the right Key Performance Indicators (KPIs) is crucial for success. These metrics provide insights into the efficiency and effectiveness of trading strategies, helping traders make informed decisions.
A. Critical KPIs for Day Trading
Average Trade Execution Time: This KPI measures the speed of trade execution. Fast execution can be crucial in day trading, where market conditions change rapidly.
Win/Loss Ratio: An essential metric that compares the number of profitable trades to unprofitable ones. A higher ratio indicates more consistent trading success.
Profitable Trade Percentage: This indicates the proportion of trades that end in profit and is key for assessing the overall effectiveness of a trading strategy.
Risk/Reward Ratio: This ratio evaluates the potential reward of a trade against its risk, guiding traders in making risk-aware trading decisions.
Maximum Drawdown: Critical for day traders, this KPI measures the largest decrease in account balance, helping in understanding the risk exposure of their trading strategies.
B. Application of KPIs in Algo Day Trading
For algorithmic day traders, these KPIs are instrumental in refining strategies:
Strategy Refinement: By assessing average trade execution time and the win/loss ratio, traders can fine-tune their entry and exit points for optimal performance.
Risk Management: The risk/reward ratio and maximum drawdown are pivotal for managing and mitigating risks, ensuring that trading strategies are not overly exposed to market volatilities.
Performance Tracking: Continuously monitoring the profitable trade percentage helps in evaluating the success of the algorithmic strategy and making necessary adjustments.
By integrating these KPIs into their routine, day traders using algorithmic strategies can maintain a balance between aggressive trading tactics and prudent risk management, setting the stage for sustainable trading success.
V. Risk Management in Algorithmic Day Trading
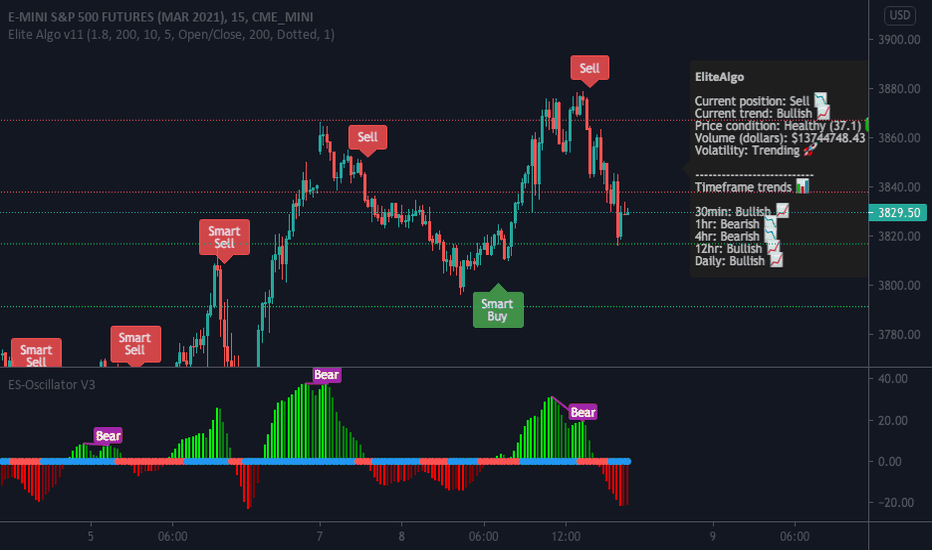
Effective risk management is pivotal in algorithmic day trading. Understanding and mitigating risks ensures the longevity and profitability of trading strategies.
A. Identifying and Managing Risks
In algorithmic day trading, risks stem from various sources:
Market Risk: This involves the risk of losses due to market volatility. Algo traders must be aware of how market fluctuations can impact their trading strategies.
Systemic Risk: The risk that a failure in one part of the financial system could cause a domino effect.
Operational Risk: Includes risks associated with technical failures, such as software glitches, connectivity issues, or system crashes.
Strategy Risk: The risk that a trading strategy may not perform as expected under different market conditions.
Understanding these risks is the first step in managing them effectively.
B. Strategies for Mitigating Risks
To mitigate these risks in algorithmic day trading, traders can employ several strategies:
Diversification: Spreading investments across different assets or strategies to reduce exposure to any single risk.
Stop-Loss Orders: Implementing stop-loss orders can help limit potential losses on a trade.
Backtesting: Testing trading algorithms against historical data to ensure they are robust under various market conditions.
Risk/Reward Assessment: Continuously assessing the risk/reward ratio of trading strategies to ensure they align with the trader’s risk appetite.
Regular Monitoring and Updates: Continuously monitoring trading systems and updating them to adapt to new market conditions and technological advancements.
By diligently applying these risk management techniques, algo traders can safeguard their strategies against unexpected market changes, ensuring a more stable and sustainable approach to day trading.
VI. Conclusion: Preparing for Success in Algo Trading

A. Integrating Learning into Practice
As we’ve navigated through the intricate world of algorithmic trading, it’s clear that integrating the insights and strategies discussed is key to success in day trading. For aspiring and seasoned traders alike, the journey doesn’t end with understanding; it truly begins with application. Putting into practice the concepts of key performance indicators, risk management, and the latest technological advancements in algo trading is crucial. This hands-on application not only solidifies your knowledge but also hones your skills, making you adept at navigating the unpredictable tides of the financial markets.
B. Continuous Learning and Adaptation
The landscape of algorithmic trading is ever-evolving, with new technologies and market trends emerging constantly. This dynamic nature of the markets calls for continuous learning and adaptation. Staying updated with the latest trends, refining trading strategies with new learnings, and being adaptable to market changes are indispensable for sustained success in algo trading. The journey in algorithmic day trading is one of perpetual growth and learning, where each day presents new opportunities and challenges to learn and excel. Embrace this journey with a mindset geared towards continuous improvement, and you will be well on your way to mastering the art of algorithmic trading.